Invited speakers
Causal inference is usually dichotomized into two categories,
experimental (Fisher, Cox, Cochran) and observational (Neyman, Rubin,
Robins, Dawid, Pearl) which, by and large, are studied separately.
Understanding reality is more demanding. Experimental and
observational studies are but two extremes of a rich spectrum of
research designs that generate the bulk of the data available in
practical, large-scale situations. In typical medical explorations,
for example, data from multiple observations and experiments are
collected, coming from distinct experimental setups, different
sampling conditions, and heterogeneous populations.
In this talk, I will introduce the data-fusion problem, which is
concerned with piecing together multiple datasets collected under
heterogeneous conditions (to be defined) so as to obtain valid answers
to queries of interest. The availability of multiple heterogeneous
datasets presents new opportunities to causal analysts since the
knowledge that can be acquired from combined data would not be
possible from any individual source alone. However, the biases that
emerge in heterogeneous environments require new analytical tools.
Some of these biases, including confounding, sampling selection, and
cross-population biases, have been addressed in isolation, largely in
restricted parametric models. I will present my work on a general,
non-parametric framework for handling these biases and, ultimately, a
theoretical solution to the problem of fusion in causal inference
tasks.
Suggested readings:
E. Bareinboim and J. Pearl. Causal inference and the Data-Fusion Problem. Proceedings of the National Academy of Sciences, 113(27): 7345-7352, 2016.
P. Hünermund, E. Bareinboim. Causal Inference and Data-Fusion in Econometrics". Columbia Causal Artificial Intelligence Laboratory, Technical Report (R-51), Dec, 2019.
S. Lee, J. Correa, E. Bareinboim. General Identifiability with Arbitrary Surrogate Experiments". In Proceedings of the 35th Conference on Uncertainty in Artificial Intelligence, 2019.
J. Correa, E. Bareinboim. General Transportability of Soft Interventions: Completeness Results. In Proceedings of the 34th Annual Conference on Neural Information Processing Systems, 2020.
E. Bareinboim, J. Correa, D. Ibeling, T. Icard. On Pearl’s Hierarchy and the Foundations of Causal Inference. In “Probabilistic and Causal Inference: The Works of Judea Pearl” (ACM Special Turing Series), forthcoming.
Elias Bareinboim is an associate professor in the Department of Computer Science and the director of the Causal Artificial Intelligence (CausalAI) Laboratory at Columbia University. His research focuses on causal and counterfactual inference and their applications to data-driven fields in the health and social sciences as well as artificial intelligence and machine learning. His work was the first to propose a general solution to the problem of ``data-fusion,'' providing practical methods for combining datasets generated under different experimental conditions and plagued with various biases. More recently, Bareinboim has been exploring the intersection of causal inference with decision-making (including reinforcement learning) and explainability (including fairness analysis). Before joining Columbia, he was an assistant professor at Purdue University and received his Ph.D. in Computer Science from the University of California, Los Angeles. Bareinboim was named one of ``AI's 10 to Watch'' by IEEE, and is a recipient of an NSF CAREER Award, the Dan David Prize Scholarship, the 2014 AAAI Outstanding Paper Award, and the 2019 UAI Best Paper Award.
Lars Peter Hansen (University of Chicago)
Uncertainty Spillovers for Markets and Policy Chair: Jasper De Bock
We live in a world filled with uncertainty. In this essay, I show that featuring this phenomenon more in economic analyses adds to our understanding of how financial markets work and how best to design prudent economic policy. This essay explores methods that allow for a broader conceptualization of uncertainty than is typical in economic investigations. These methods draw on insights from decision theory to engage in uncertainty quantification and sensitivity analysis. Uncertainty quantification in economics differs from most sciences because there is uncertainty both from the perspective of an external observer and from people and enterprises within the model. I illustrate these methods in two example economies in which the understanding of long-term growth is limited. One example looks at uncertainty ramifications for fluctuations in financial markets, and the other considers the prudent design of policy when the quantitative magnitude of climate change and its impact on economic opportunities is unknown.
Additional information
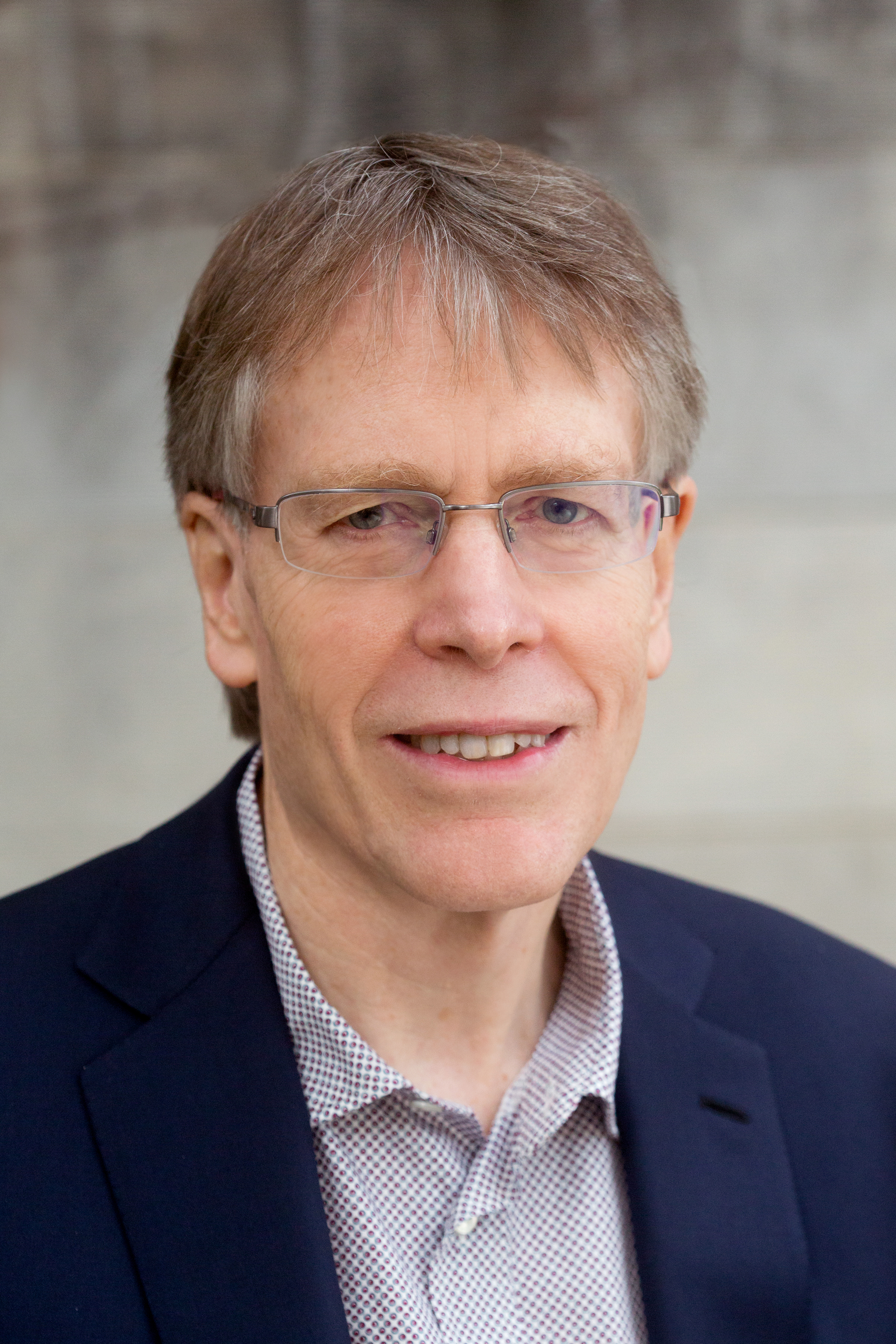
Lars Peter Hansen is the David Rockefeller Distinguished Service Professor and the Director of BFI’s Macro Finance Research (MFR) Program at the University of Chicago. He is the leading expert in economic dynamics who works at the forefront of economic thinking and modeling, drawing approaches from macroeconomics, finance, and statistics. Hansen has made fundamental advances in our understanding of how economic agents cope with changing and risky environments. He is the recipient of the 2013 Sveriges Riksbank Prize in Economic Sciences in Memory of Alfred Nobel.
Marco Zaffalon ("Dalle Molle” Institute for AI)
Imprecise probability in causal-AI wonderland Chair: Enrique MirandaIs there a role for imprecise probability in the new world dominated by Artificial Intelligence and Machine Learning? Shall we stick to a logical-based view of imprecise probability, acting as purists of truth and doing great theory, while having a little impact on applications? Or shall we find a compromise between behaviouralism and the pragmatism needed to have an impact in Statistics. Is radical epistemic probability paying out. Or there is room for some kind of physical probability with tighter connections to the world. And what about the new research strand of causal inference; is it saying anything about us? I believe it is saying much, and that we should take causal inference seriously. In this talk I will discuss these ideas as well as the connection between imprecise probability and causal inference. I argue that there is a strong link between the two, but that there are also sources of disagreement, which we should face as a community.
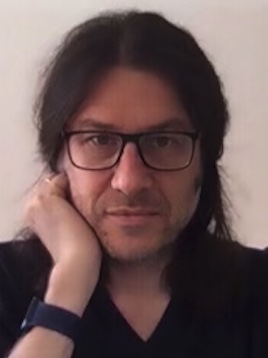
Marco Zaffalon is a Scientific Director of the "Dalle Molle” Institute for AI (IDSIA.ch, USI-SUPSI) at Lugano, Switzerland, and is a Professor at SUPSI.ch. Marco has a PhD in applied mathematics and a long history of research in imprecise probability, ranging from credal classification and credal networks to desirability and subjects with more of a foundational flavour. His recent interests are in causal inference. At IDSIA he leads a group of 30 researchers, many of whom with an interest in imprecise probability. A year ago Marco co-founded Artificialy.com, which is a startup for products and services in AI, where he has the role of Chief Scientist.
News
Important dates
- Submission deadlines:
Long and short papers: February 10th, 2021.Title and abstract: February 20th, 2021.Full paper: February 28th, 2021.1-page abstracts: April 20th, 2021.Poster abstracts: May 15th, 2021.- Poster abstracts final version: June 15th, 2021.
- Upload poster files to Researchers.One: June 28th, 2021.
Paper notification: April 15th, 2021.Paper final version due: May 10th, 2021.
Organisation
ISIPTA 2021 is organised by the UTAI group, a research group of the Department of Computer Science and Artificial Intelligence, University of Granada.
For info about the conference, contact isipta@decsai.ugr.es. For comments about the website, contact Manuel Gómez-Olmedo.